CASE STUDY:
BAYER’S "FUTURE CLINICAL TRIALS"
PROJECT UTILIZES
VEIL.AI'S NEXT-GENERATION ANONYMIZATION
This case study demonstrates:
- How anonymized data can be of such a high quality that the same conclusions can be drawn from it, as from traditional pseudonymized, individual-level research data
- How sensitive data can be anonymized, after which it can be transferred to another country to be enriched and further utilized
- How anonymization enables reuse of organization’s Legacy data
- What were the steps to build a synthetic control arm for a clinical trial
This is a significant achievement. In our study, we could draw the same conclusions from anonymized data as from traditional pseudonymized, individual-level research data.
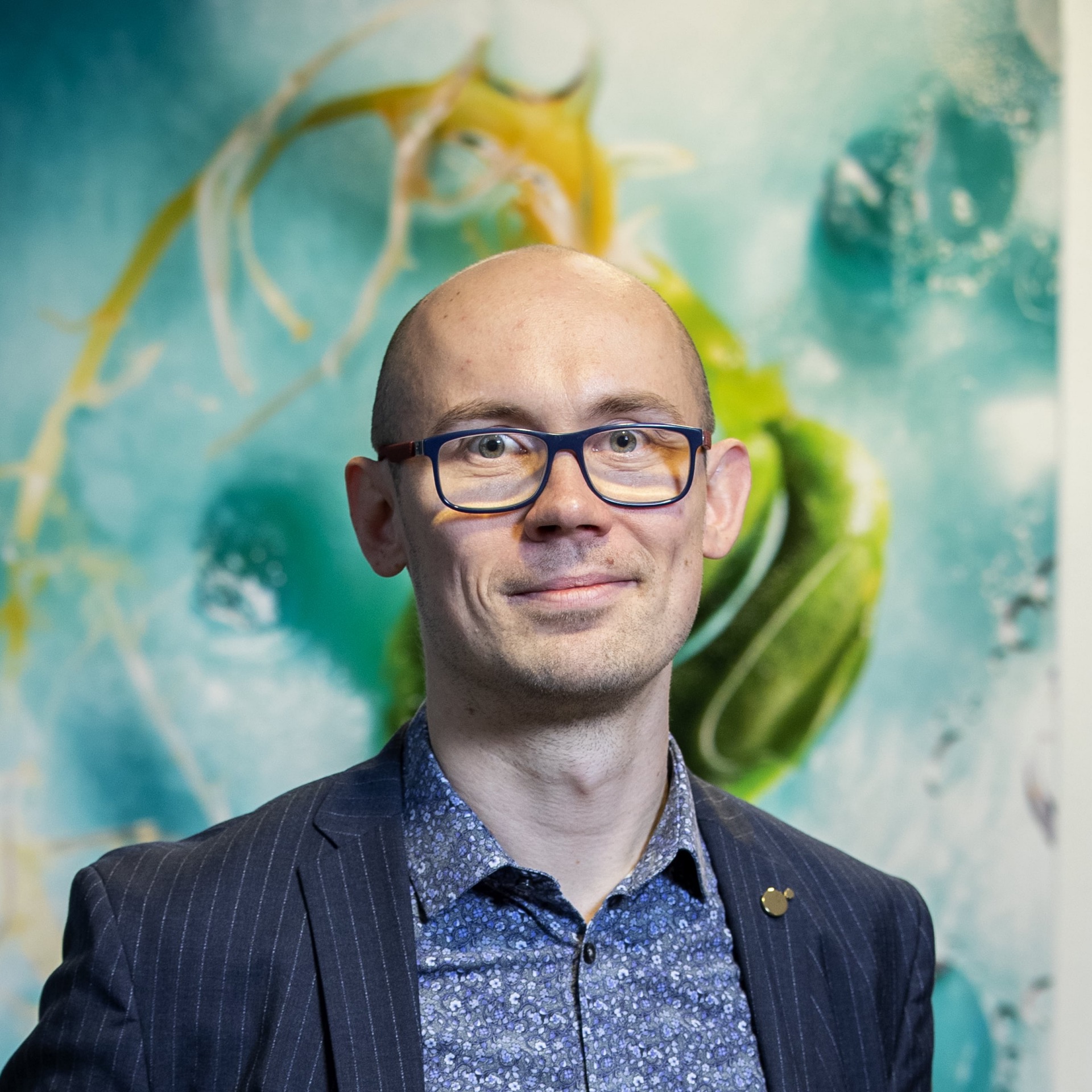
Jussi Leinonen,
Strategic Project Lead
Bayer

BACKGROUND AND TARGETS
Drug development is a laborious, time-consuming process. On average, developing a new drug takes 10 years – about seven of which are spent on clinical research. This does not even touch on the considerable costs of developing a new prescription drug, which can amount to two billion euros on average.
Global life science company Bayer carried out a three-year multi-million-euro development project called Future Clinical Trials (FCT), which utilized AI-enhanced next-generation anonymization technology from VEIL.AI.
The international project was aiming to find ways to:
speed up clinical trials
reduce drug’s time to market
increase patient safety
reduce costs
VEIL.AI BECOMES BAYER’S ANONYMIZATION PARTNER
CONCEPT FOR ANONYMIZING INTERNAL LEGACY DATA
The created concept document gave the Data Scientists and the project team support to promote a new kind of thinking internally. It helped them to explain that RCT data is often pseudonymous data with limitations, and it cannot be freely reused. By anonymizing the internal dataset, the data becomes GDPR-free and reusable for the organization providing great new use cases and increasing the value of the data. The greatest value of enabling efficient data sharing is that collaboration and co-development with external collaborators is much easier.
For Bayer's international organization it was also important to show the differences in regulations e.g., that anonymized data has stronger privacy protection than HIPAA de-identified data. From a European point of view, HIPAA compliant data does not fulfill the definition of GDPR anonymized data, but is closer to pseudonymized data since it may include identifiable people. Therefore, HIPAA de-identified data is most likely restricted by GDPR and that is why in Europe Bayer needed to go beyond the HIPAA regulation.
WHY DOES BAYER NEED ANONYMIZED, PRIVACY COMPLIANT REAL-WORLD EVIDENCE & RANDOMIZED CLINICAL TRIAL DATA?
Bayer was concentrating on two focus areas:
Building an external / synthetic control arm for clinical trials
Internal data re-use and collaboration with external partners
WHY A SYNTHETIC CONTROL ARM WITH ANONYMIZED DATA?
Patient recruitment and retention in clinical trials are major challenges in the development of new drugs. If there is high-quality anonymized data available, part of the control patient group could be replaced in some instances with an external control arm – i.e., with a group formed based on external health data, most commonly data from real-world data like electronic health records, registries or previous clinical trials.
This can have many positive impacts like increasing efficiency, reducing delays, speeding up a drug's time-to-market and lowering costs in the evaluation of new therapies.
Bayer also wanted to build the capabilities for embedding RWD into clinical development programs. The idea was to anonymize the pseudonymized external RWD, which enables the data to be transferred to Bayer’s own environment, where the anonymized data could be merged with Bayer’s own anonymized legacy RCT data.
RE-USE OF BAYER’S LEGACY DATA WITH EXTERNAL PARTNERS
Another big topic was how to enable efficient data sharing and re-use of Bayer's own legacy RCT data with external partners. Re-use of legacy data provides huge opportunities and its value can increase drastically.
If the data is anonymized with high quality it can naturally also be re-used internally and in addition, collaboration and co-development with external partners is much easier.
In the FCT project, indication specific harmonized and curated datasets were created in Bayer’s (Microsoft Azure Databricks) data science environment. VEIL.AI's AI-enhanced next-generation anonymization technology enabled the re-use of legacy data. The anonymization results were excellent.
If legacy data is anonymized, an external partner can be granted access to the data on organization’s own server without having to send the data anywhere (outside the organization).
Anonymization of Bayer Internal data
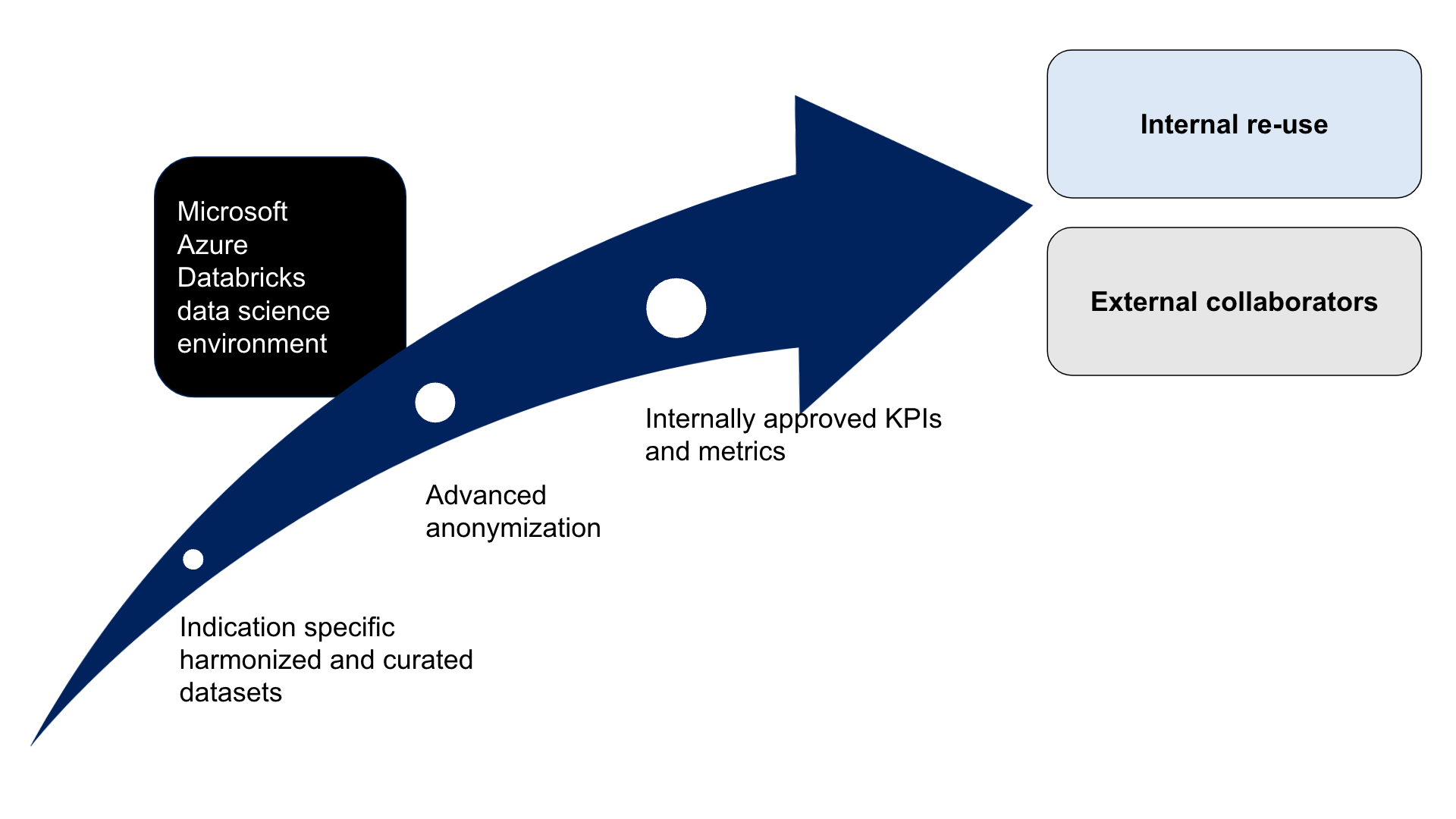
STEPS TO BUILD AN EXTERNAL CONTROL ARM
DATA FROM REGISTER HOLDERS
When talking about external control arms, Bayer wanted to create a methodology for providing virtual or synthetic controls for clinical trials based on RWD and legacy clinical trial data.
The FCT project was implemented in Finland, which has good health data registers and the first legislation for secondary use of health data introduced in Europe. The name of the health data permit authority is Findata.
The new data needed for Bayer's external control group was collected from national registers and databases directly into Findata's secure operating environment, where it was cleaned, checked and processed.
HIGH-QUALITY DATA ANONYMIZATION
After that VEIL.AI anonymized the pseudonymized data with next-generation anonymization technology with very high quality. In fact, the next-generation anonymized data was of such high quality that the same conclusions could be drawn from it as from traditional, pseudonymous individual-level research data (read more about the published study article here).
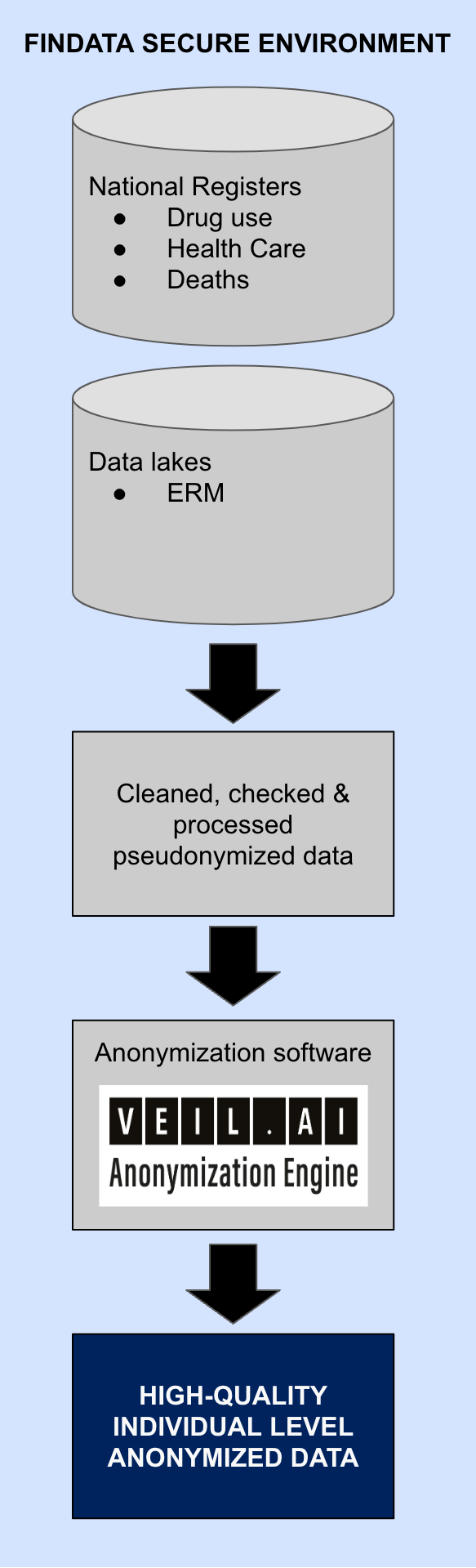
VERIFICATION OF ANONYMITY BEFORE DATA RELEASE
So now Bayer had new high-quality anonymized register data in Findata's data secure environment. But how can an authority or a Data Privacy Officer (DPO) know that an anonymized dataset is really anonymous? How can anonymity be verified before anonymous data is released to be transferred to another country?
VEIL.AI CREATES A PROTOCOL TO VERIFY ANONYMITY
VEIL.AI has also solved this issue; VEIL.AI has created a protocol with which authorities and DPO’s can verify the anonymity of datasets that have gone through the anonymization process. The analysis is a transparent way to provide strong evidence of privacy protection before releasing the anonymized data for further use and helps to document what has been done during the anonymization process.
AUTHORITY CONSENTS TO THE TRANSFER OF ANONYMIZED DATA TO ANOTHER COUNTRY
After Findata was able to verify the anonymity of the dataset, it gave consent for Bayer to export the verified anonymous data to Bayer’s own secure data science environment.
- merging the anonymized data with legacy RCT data
- getting more anonymized RWD and RCT data
- data augmentation to extend the follow-up time and improve the efficiency of clinical trials
NEXT-GENERATION ANONYMIZATION & PERSPECTIVES FOR THE FUTURE
Bayer identified various different use case opportunities for anonymization, related to both focus areas (real-world-evidence and randomized clinical trials).
The aim is to move towards defined standards and continuous anonymization capability included in data science platforms and workflows.
Data Scientists in data driven organizations need good data to practice high-quality data science. Continuous next-generation anonymization capability can help to do things that would otherwise not be possible.
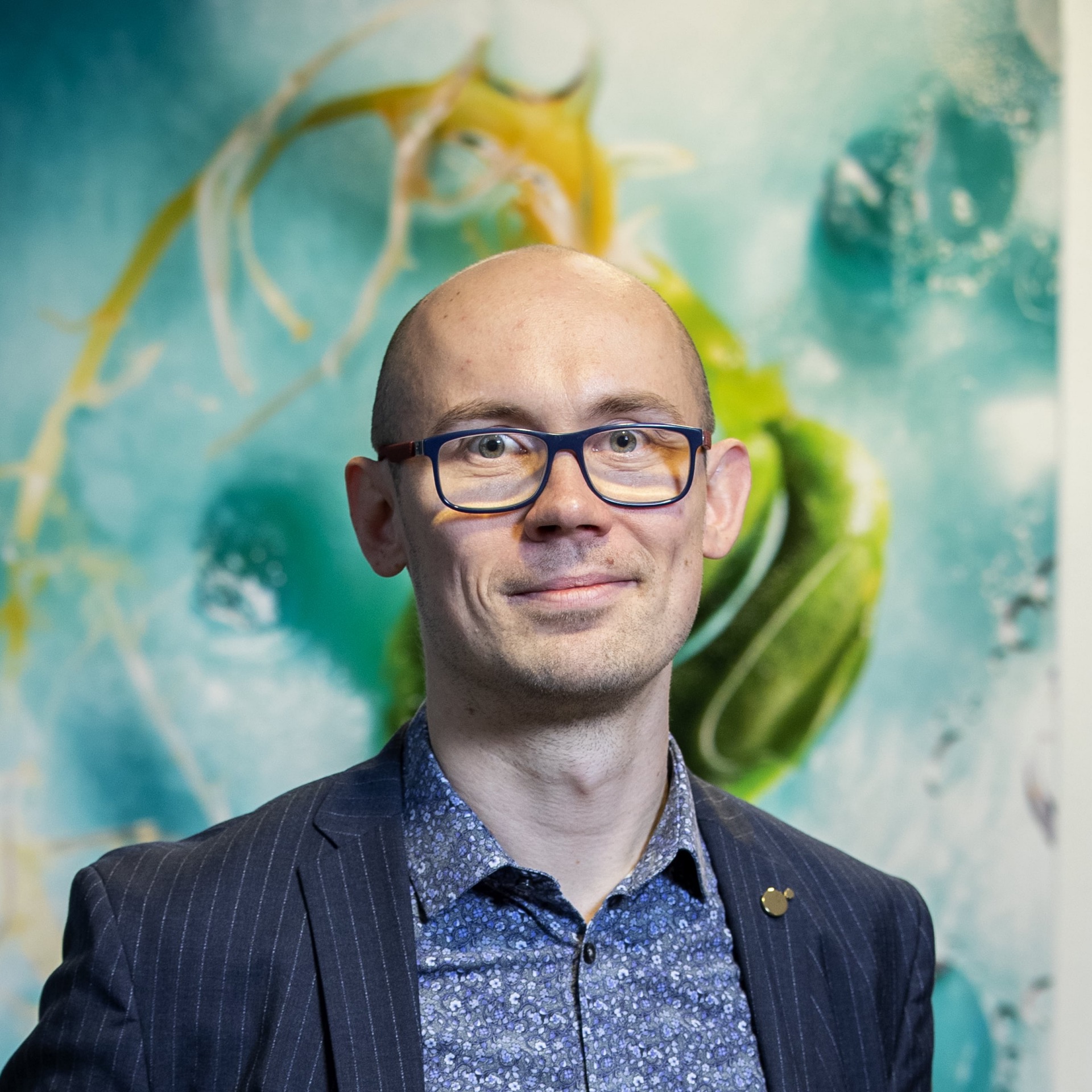
Jussi Leinonen,
Strategic Project Lead
Bayer

Want to to discuss your use case with us?
Are you interested in our services and solutions? Let’s start a conversation about how we could help you.
*By submitting this form you agree to our Privacy policy. We are committed to your privacy. Information provided by you may be collected and automatically stored in our database and may be used for sending you additional information about VEIL.AI and our services. You may unsubscribe from these communications at any time.