Following the passage of the European General Data Protection Regulation (GDPR) in 2018, there has been much confusion between privacy regulations in the EU and the United States. Let’s take a look at the difference between US de-identified data and EU anonymized data.
What is de-identified data?
De-identified data is defined by the 1996 Health Insurance Portability and Accountability Act as
“Health information that does not identify an individual and with respect to which there is no reasonable basis to believe that the information can be used to identify an individual”
In plain English, de-identified data applies only to identifying information as part of protected health information. De-identification is reversible if the entity de-identifying the data creates a key matching masked values to direct identifiers.
What is anonymized data?
Anonymized data is defined in the EU GDPR as
“…information which does not relate to an identified or identifiable natural person or to personal data rendered anonymous in such a manner that the data subject is not or no longer identifiable.”
In other words, anonymization is an irreversible process where an individual can’t be re-identified. That means there is not a key anywhere matching direct identifiers to masked values, available somewhere to some individuals, and that there is not enough information within the dataset or that can be combined with the dataset to re-identify an individual.
Can HIPAA de-identified data be GDPR anonymous data?
Whether HIPAA de-identified data is considered anonymous by GDPR standards depends on the method that was used to de-identify the data. HIPAA offers two routes to de-identify protected health information:
Expert Determination
Expert determination (HIPAA §164.514(b)(1)) is when an expert designates the data as de-identified. It involves a very small risk of identification, but is often time consuming and usually results in the loss of useful data.
Safe Harbor Method
The safe harbor method (HIPAA §164.514(b)(2)) involves the removal of 18 known direct identifiers (including name, zip code, birth date, and social security number, among others) from the data and certifying that the entity de-identifying the data does not have actual knowledge that the information can be used alone or with other information to re-identify an individual in the dataset.
For de-identification purposes, actual knowledge means clear and direct knowledge that the de-identified information could be used, alone or in combination with other data, to re-identify an individual in the dataset. This clear and direct knowledge means, for example, that an individual in the dataset has a revealing occupation, that there is a clear familial relation between the recipient of the data and a data subject, a publicized clinical event (like giving birth to eight children), or any other knowledge that the recipient of the data could re-identify an individual.
What it does not mean, however, is knowledge of research or methods in re-identifying individuals in de-identified data in general, however.
The safe harbor method is fast, cost-efficient, and keeps more useful data for analysis. Yet because of the method of de-identification, it is not likely that the data is considered anonymized under EU GDPR regulations.
Examples of a de-identified dataset that is not GDPR anonymous
Let’s say we have a dataset from a hospital that has been de-identified. The hospital records can’t include direct identifiers in the dataset, but they include a large amount of information on hospital procedures, including rare clinical events. Even if the hospital records don’t include dates of specific diagnoses or treatments for individual patients, we know that the records cover only a specific period of time.
For example, let’s say the hospital dataset contains a record of a patient diagnosed with a rare disease related to a mutation in one specific gene. If the diagnosis is rare enough, the patient might be the only person in the entire dataset with that specific diagnosis. And while the diagnosis might not be sensational enough to be a publicized clinical event, it’s entirely possible that the patient themselves might have spoken publicly about their diagnosis or posted about it in public on social media. Because there are individual records in the de-identified dataset that are sufficiently unique, there is the possibility for the person receiving the data to combine it with other information to re-identify an individual person, even if the entity de-identifying the data is not aware of it. This would be sufficient to mean that the data is not considered GDPR anonymous.
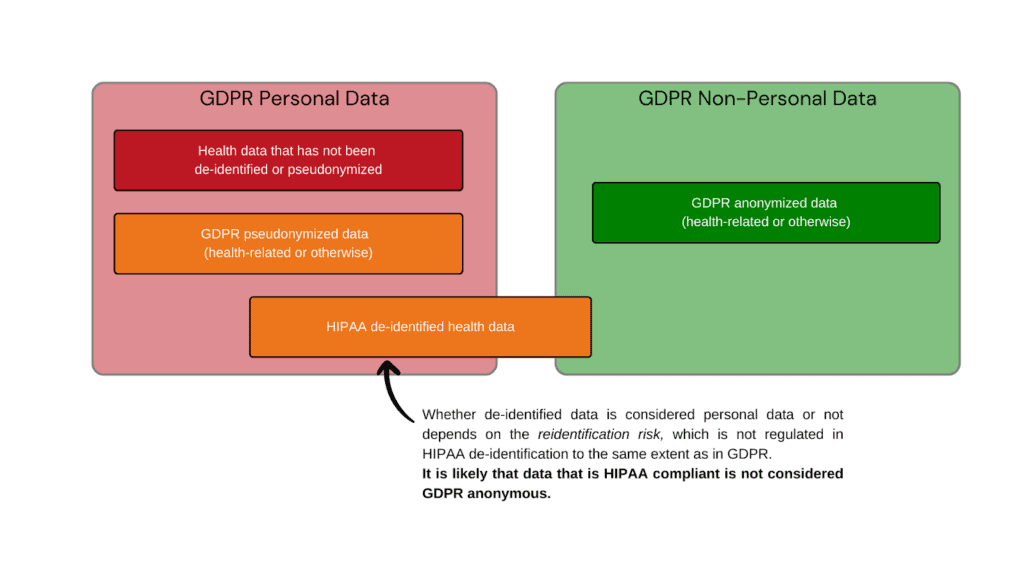
VEIL.AI can help you ensure your dataset is GDPR compliant
VEIL.AI’s next-generation anonymization can serve two purposes to ensure that your dataset is not just HIPAA compliant, but GDPR compliant.
First, VEIL.AI’s technology provides a privacy risk assessment analysis of your data, evaluating whether your data contains any GDPR personal data and if it would be considered GDPR compliant. VEIL.AI’s state-of-the-art privacy risk analysis quantitatively evaluates privacy in a way that is compliant and in line with the GDPR regulations.
Secondly, VEIL.AI’s technology can be used to anonymize datasets to guarantee that they are GDPR compliant. Other anonymization technologies, much like the HIPAA expert determination of privacy, can be slow, expensive, and produce low quality data. The VEIL.AI Anonymization Engine is fast and produces anonymized data that is highly similar to the original data and extremely useful for downstream analyses.
Want to find out more about how VEIL.AI can help you achieve GDPR compliance?