“Medicine is no longer a clinical science just supported by data; it’s moving to a field defined as clinical science in collaboration with data science. Patient data is one of the most important drivers of this change”. -Ernst & Young*
The biggest obstacle to achieve the world as described in the above quote is NOT the existence and quality of data. Data already exists in different hospital or clinical data lakes, registries and other repositories. And societies have invested trillions of euros and tens of years to generate the data. The problem is how to get access to the data and how to harmonize it in such a way that different data sources can be combined. In this blog post I will focus on the first of these questions, i.e. how to improve access to data.
Problems accessing sensitive data can be solved with anonymization
The biggest problem as far as access is concerned is privacy. Health data is sensitive - it contains information about private people. Sharing, pooling and use of the data is highly regulated. Anonymization has been touted as a solution to the problem of getting access to larger and more high-quality data sources for health data analytics, but what does it really mean? How is it different from pseudonymization, and what does it all mean in terms of privacy legislation?
How is anonymization different from pseudonymization?
Pseudonymization is a term defined in the European General Data Protection Regulation (GDPR), which simply means the removal of direct identifiers from a dataset. Importantly, this is a reversible process- information can still be connected to an individual person, by using traceable information in the data to infer the identity of a single person. Pseudonymization is a widely used method, and it actually is highly useful in preventing the most common violation of privacy- inappropriate access to data records by authorized users (for example, a doctor looking up health records for a celebrity patient being treated in their hospital).
Why is pseudonymized data subject to GDPR?
The problem with pseudonymization is that even if the direct identifiers connected to the pseudonymized data are destroyed, the data may still contain information that can uniquely identify a single individual. If you are a healthcare provider treating a patient whose occupation is ‘President of the Republic of Finland’, you would know quite well what the identity of the patient was, even if the data has no direct identifiers like name or social security number. Because of this potential to uniquely identify individuals in pseudonymized data, it is treated under GDPR legislation as personal data, and protected with the same laws and rules as data that actually contain direct identifiers like names.
This results in what has been called the silo problem- useful data are walled within institutions and countries, with restrictions in processing and transfer, resulting in:
- Access to data taking tremendous amounts of time
- Lack of interoperability of datasets
- Difficulty or impossibility of using pooled data to investigate, e.g., rare diseases or endpoints
- Inability to use data beyond research permit specifications (e.g. innovation and development, or any other secondary use not defined in the original research permit)
How can anonymized data solve the silo problem?
Anonymized data, on the other hand, is defined in GDPR as data that can’t be reasonably used to re-identify an individual in the dataset. This is an irreversible process, where the data can’t be linked to the original subjects after the anonymization is complete. Because anonymized data can’t be connected to a person, it is not considered personal data under GDPR. This means that the data can be shared freely- because it is not restricted under privacy legislation, and because the data is safe from the threat of privacy violations.
How does anonymization actually work? It involves altering the data, for example by
- Binning variables, like changing age: 27 to age: 25-30
- Removing variables that are too revealing, like postal code
- Removing individual persons in the dataset, like the President of the Republic’s records and many other methods. A complete list of usually applied anonymization techniques are listed below.
Suppression | Deletes values or records |
Generalization | Diminishes granularity of the data by binning, reducing specificity |
Noise addition | Adds noise to the data; works well with continuous numerical data |
Data swapping | Moves values between records; difficult to do without changing multivariate distributions |
Synthetic data insertion | Adds statistically neutral dummy records to the data |
So if anonymized data can solve the silo problem and unleash the power of data, why isn’t everyone already using it for all data applications?
Why isn’t anonymization widely used already?
The problem is that the current state of the art methods in anonymization have two main drawbacks: first, anonymization methods often remove many of the most interesting parts of the data that are relevant for analysis, throwing the baby out with the bathwater and making the data less useful for data analysis. This is because in the existing anonymization techniques it is tedious to control and manage which variables are more important than others and what kind of data transformation methods are preferable. In other words, it is hard to control the information loss during the anonymization process. Second, the methods of anonymization are often manual or with limited automation, making them slow and expensive options. In all, data anonymization thus far has not been a good enough tool for solving the silo problem.
VEIL.AI’s Anonymization Engine outperforms existing methods
VEIL.AI’s Anonymization Engine, however, is a head above when it comes to results. There are a lot of different ways to anonymize a dataset, and manual or simple automation of the process is not likely to give the optimal solution. VEIL.AI’s Anonymization Engine utilizes neural networks to find the best way to alter the data to give the perfect balance of privacy and data utility in the anonymized data. The interesting parts of the data are kept in, and the resulting data is highly useful for analysis, while the risk of re-identifying an individual in the data is extremely low**.
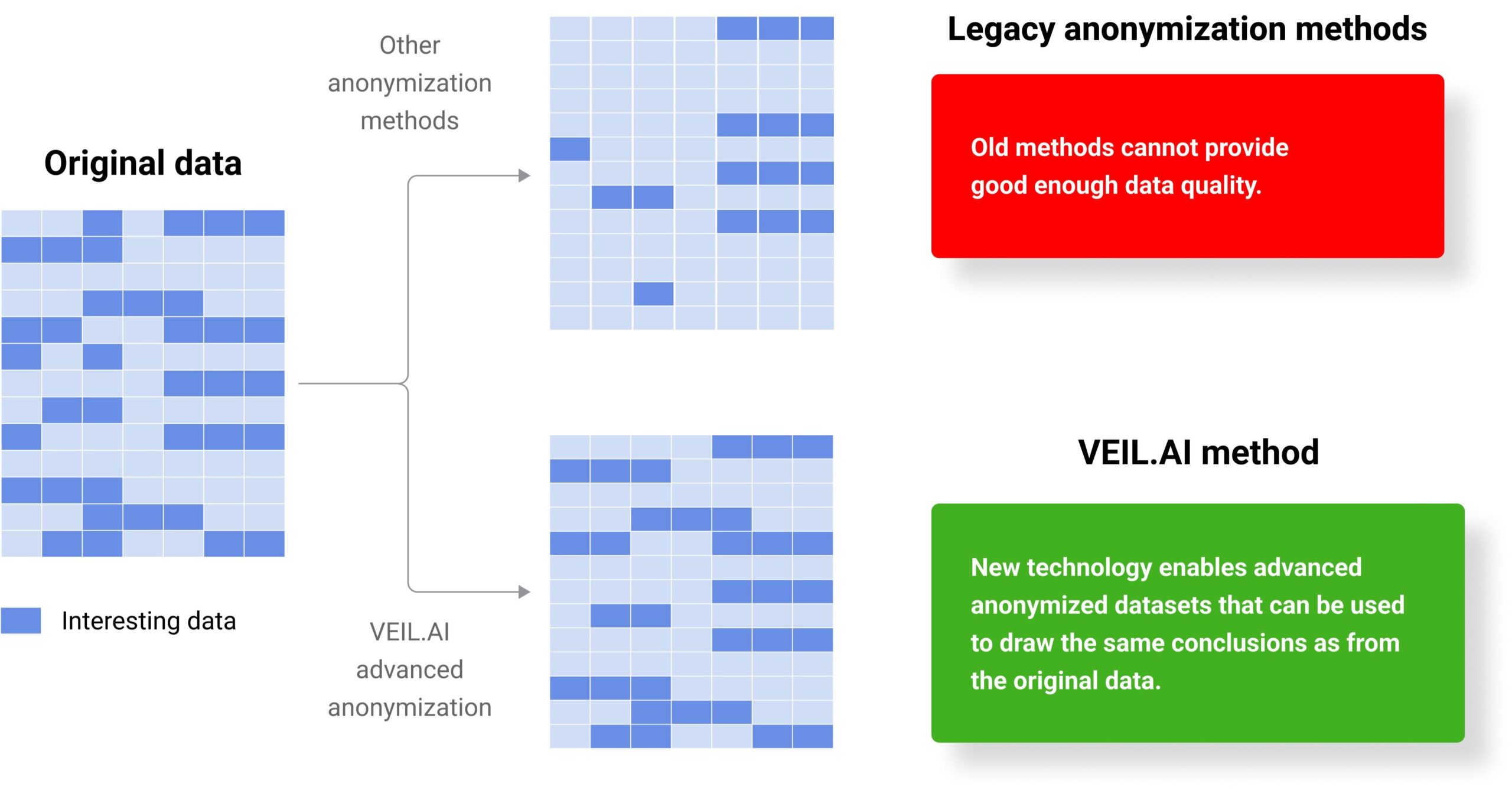
By using VEIL.AI’s Anonymization Engine to produce shareable, GDPR-free datasets that are of extremely high quality and utility, we can solve the silo problem and unleash the power of data for healthcare, analytics, and the betterment of society through data. Join us in our journey to transform health data.
____________________
*https://assets.ey.com/content/dam/ey-sites/ey-com/en_gl/topics/life-sciences/life-sciences-pdfs/ey-value-of-health-care-data-v20-final.pdf
** There are good gold standards to what kind of residual risk is acceptable. Good readings are e.g. https://www.privacy-regulation.eu/en/recital-26-GDPR.htm or https://www.ema.europa.eu/en/documents/regulatory-procedural-guideline/external-guidance-implementation-european-medicines-agency-policy-publication-clinical-data_en-3.pdf