Why is integrating health data from multiple sources important?
The future of medicine is built on data, with an emphasis on novel methods of analyzing and utilizing the data like machine learning. Some of the most frequent use cases and needs concerning data use that pop up in discussions with our customers include more data for Real World Evidence (RWE) or clinical trials. Very frequently, customers also ask for more value from data insights and faster access to data (see a list of common benefits from data that customers are seeking in the table below).
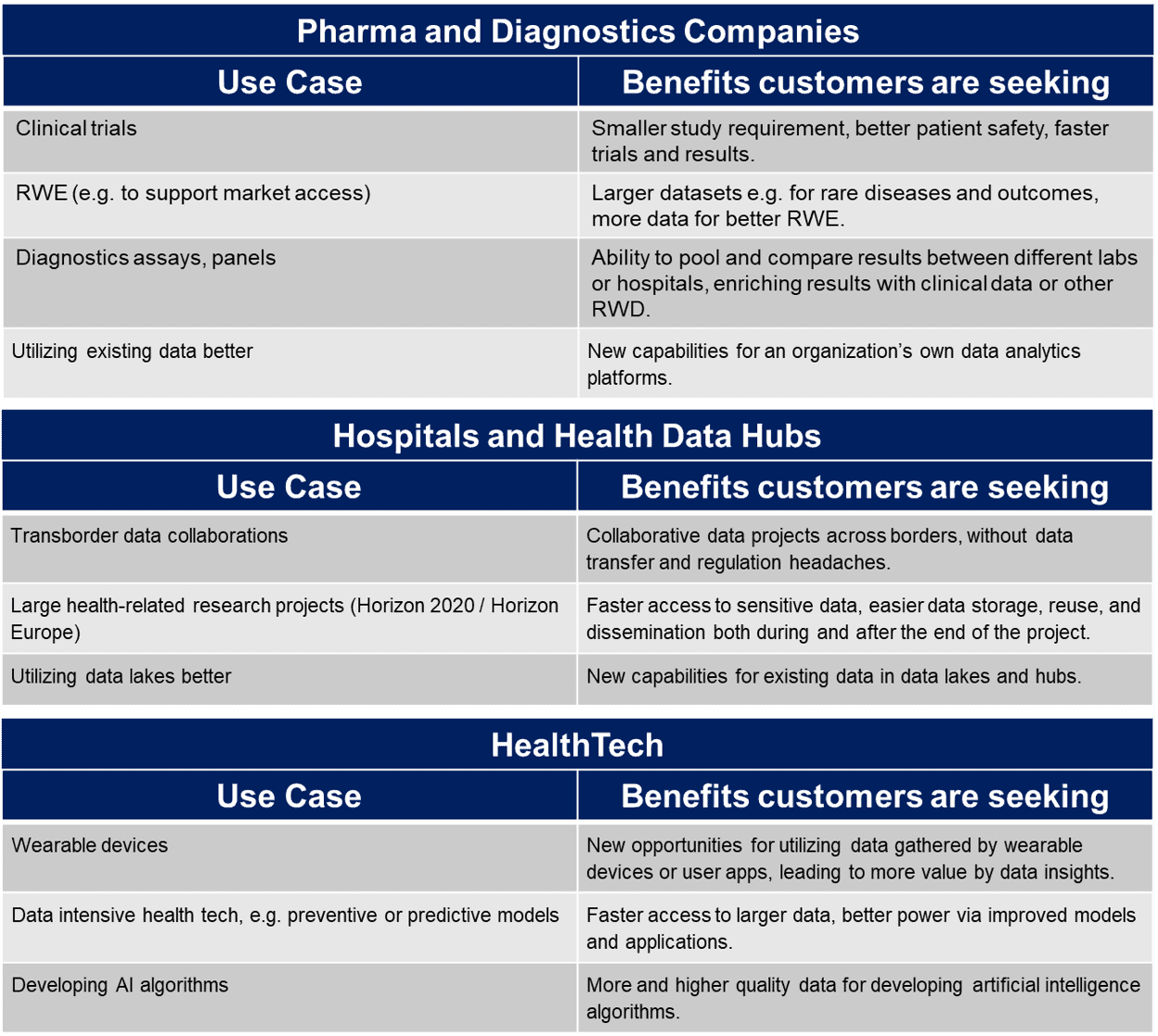
These benefits can often be found by combining data from multiple sources to create larger data pools (or jointly analyzing data in existing data hubs or lakes). But what are the ways that data are currently combined from multiple sources, and what are the pitfalls of these methods?
What are the current approaches for combining data?
Sharing data
Permit processes make data sharing slow, there are plenty of privacy risks involved, and legislation restricts the possibility of sharing the sensitive data. Reuse is also difficult, as laws like GDPR stipulate that data use must be defined carefully, and when the reason for data access ends the data must be deleted or returned.
Trusted Third Party
Using a trusted third party is still very slow due to permit processes, and may lead to a situation where data users cannot affect nor see what is done to the data. Similar to direct data sharing, data use is still strictly defined for a particular use case.
Federated Learning and Data Approaches
Federated approaches like federated learning work in situations when it is hard to move the data and form large data pools. Instead of moving the data, the alternative is to let the algorithms move instead. Analysis is made at each data source, and a common, robust machine learning model is generated without sharing data. Federated learning is a very useful approach, but there are still privacy risks involved if the learning is done on original, sensitive data. This means that from a privacy protection perspective, federation alone does not solve privacy issues. Anonymization and federated learning are actually complementary, where data should be first anonymized and only then used for federated learning.
Synthetic Data
Synthetic data is an approach that, by being free of identifying information, bypasses privacy legislation and requirements. Yet often synthetic data has too little power, especially when used for longitudinal analysis and/or complex research questions.
Old Anonymization Methods
Older anonymization methods create data that satisfies privacy regulations, but much of the interesting data is lost. The resulting dataset is low quality and unsuitable for downstream analysis.
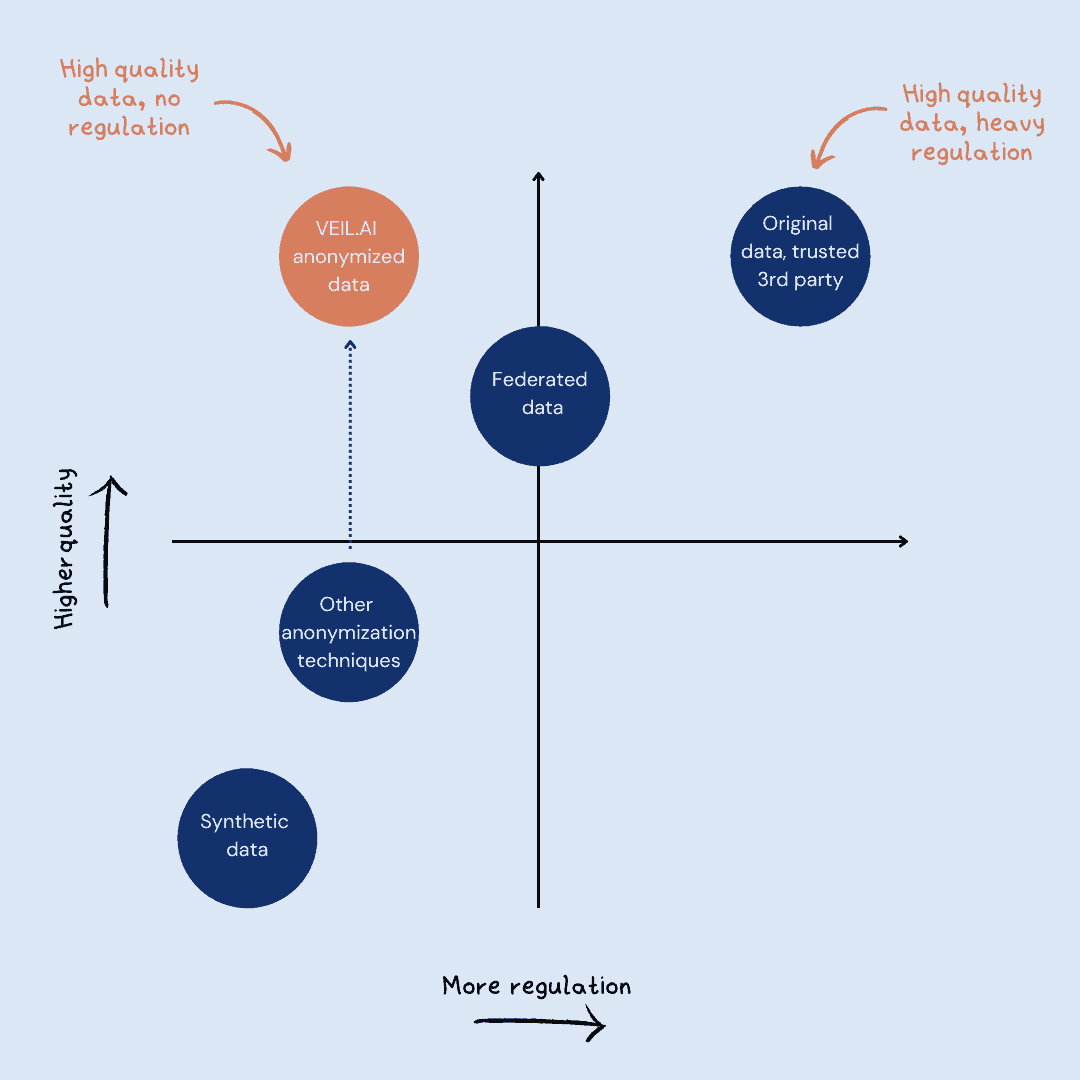
How VEIL.AI advanced multiparty anonymization is different?
Anonymized data is by definition data without privacy risks or personally-identifying information, so GDPR and other privacy legislation do not apply to it. As anonymization for data that needs to be combined is done “at the source”, you never share sensitive data and compliant data sharing is exponentially simplified. Storage and data reuse for different purposes is also much easier, as this is unrestricted in the combined, anonymized dataset.
VEIL.AI’s Advanced Anonymization is also capable of supporting and anonymizing rapidly accumulating data types, such as from wearable devices. And with VEIL.AI’s unique technological solution, the resulting data quality and utility is very high – VEIL.AI anonymized data looks like, behaves like, and has the statistical power of the original data.
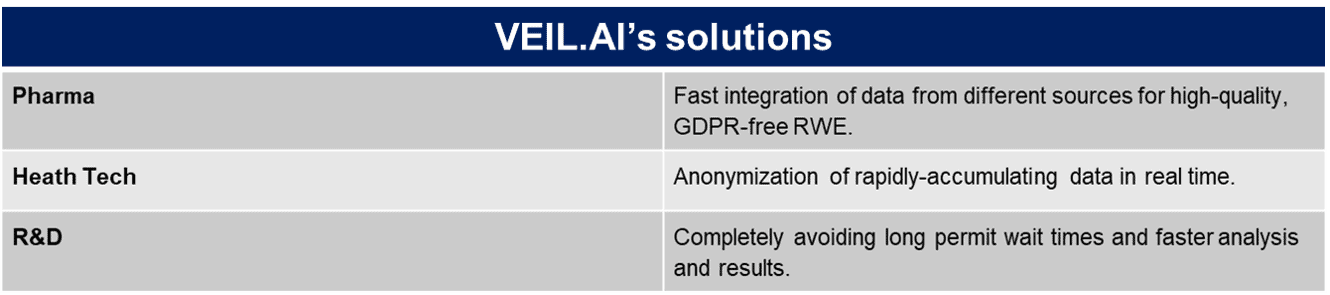
How is multiparty anonymization done in practice?
VEIL.AI’s multiparty anonymization is accomplished by deploying the anonymization model to each source - for example, as a functionality of their data lake. The data anonymization is done ‘at the source’ and data analytics are performed in a designated access-controlled and secure collaboration environment, like the VEIL.AI Data Collaboration Platform. After the anonymization is complete, the data no longer contains personally-identifying information- meaning it does not have privacy risks, is free from privacy legislation, and can be freely combined!
Want to find out more about VEIL.AI’s solution for generating all the high-quality data you need for your use case in a fast, hassle-free way? Contact [email protected] (tel. +358406646300) to set up a meeting to discuss more.